The surface soil moisture state can be inferred by using remote sensing data from Multi-Wavelength Satellite Observations in a process known as retrieval. Neural Network Soil Moisture Retrieval is able to provide global soil moisture estimates at daily or sub-daily resolution.
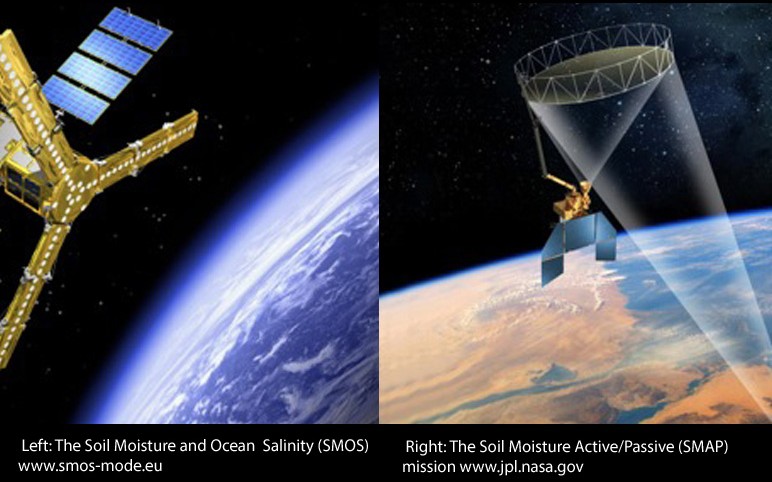
When considering the Earth’s total water budget, soil moisture – that is the water content of the soil – constitutes only a minute part (about 0.005%). Nevertheless, this parameter has been largely recognized as one of the key parameters in the study of the Earth system and has even been named as one of the Global Climate Observing System (GCOS*) Essential Climate Variables. While at first sight it may be surprising that so small a parameter is attributed such importance, this decision is explained by the fact that the soil water content is at the boundary between the land surface and the atmosphere and as such strongly drives interactions between these two. This is mainly because the soil moisture state impacts the soil evaporation and plant transpiration as well as the surface sensible heat flux. Sensible heat flux is transference of heat energy from the Earth’s surface to the atmosphere by conduction and convection. Atmospheric circulation can then move this heat energy horizontally.
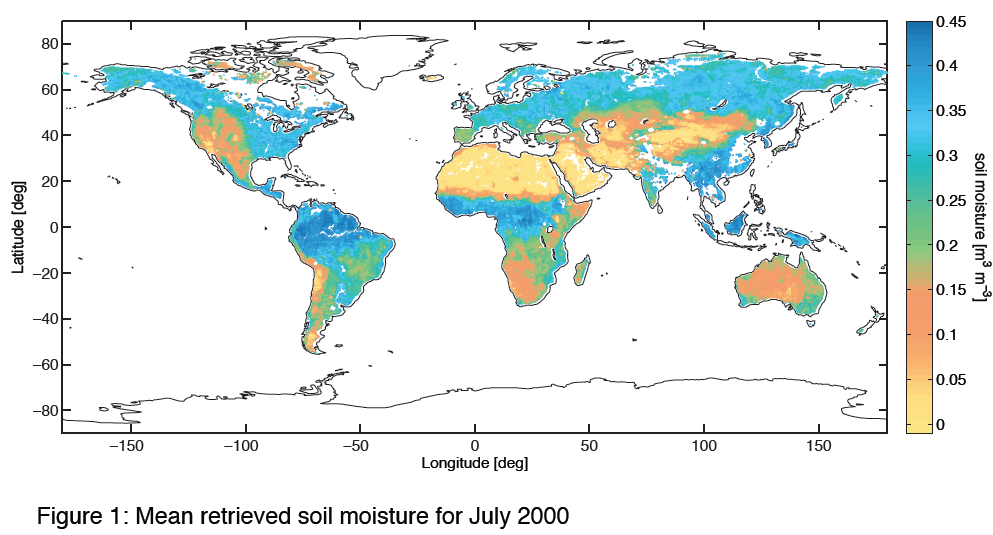
The influence of soil moisture on land-atmosphere interactions makes it a key parameter in the development of numerical weather prediction (NWP) and Earth System Models (ESM) that are used to predict the weather or the future Earth climate. This is because a good soil moisture parameterization makes it possible to correctly predict the atmospheric motion, the temperature response of the surface or the CO2 uptake of plants, among others. While the importance for weather and climate modeling feature among the main reasons for a strong interest in soil moisture, other domains are equally affected. For example, the water content of the soil is of great concern for many agricultural applications, since it allows farmers to monitor plant health, predict crop yields and plan more precise irrigation schedules. Soil moisture equally affects the partitioning of precipitation into infiltration and surface runoff and is thus of interest for water resource management, pollution control, and the study of surface erosion. Furthermore, the soil water content is of great importance for fire, flood and drought risk assessment. A good understanding of soil moisture and its governing processes is essential for many applications.
To fulfill this demand for soil moisture data, large networks of in-situ stations have been established to provide ground measurements of soil moisture. However, many of the aforementioned applications require global soil moisture observations with a finer temporal resolution (daily or less)—which cannot be met by the current networks. The surface soil moisture state can be inferred by using remote sensing data from a set of satellite observations in a process known as retrieval. Satellite retrievals are able to provide global soil moisture estimates at daily or sub-daily resolution. The resulting data sets are ideal for applications such as the development and evaluation of land surface models or global studies of hydrological processes.
Traditionally, retrievals are performed through the inversion of Radiative Transfer Models (RTMs), which are explicit formulations of the physical processes relating the geophysical parameter of interest (soil moisture) to the satellite observation. In the case of soil moisture, these RTMs are highly complex and require the use of considerable amounts of a priori information. Our work thus focuses on the development of alternative soil moisture retrieval methods with the aim of reducing the retrieval uncertainty and providing better quality soil moisture products. The primary focus relies on the development of statistical retrieval algorithms and in particular the use of artificial neural networks. Their ability to capture the statistical links between satellite observations and soil moisture eliminates the need for explicit physical formulations and the use of a priori information. Additionally, neural networks facilitate the combination of different satellite data to fully exploit the synergy of different sensors. This layering technique was used to create a combined soil moisture product from a combination of multi-wavelength satellite sensors (active and passive microwave, infrared and visible) not dedicated to soil moisture observations. A global, monthly soil moisture retrieval dataset has been generated for the period 1993-2000, and a daily dataset for the period 2002-present will be made available soon. However, the neural network retrieval technique is equally appropriate for dedicated soil moisture missions, such as the Soil Moisture and Ocean Salinity (SMOS) mission or the upcoming Soil Moisture Active/Passive (SMAP) mission. Currently, a neural network-based retrieval algorithm is being developed for SMAP data, which aims to fully exploit the information on different soil moisture variations provided by the radiometer and radar carried by SMAP. In addition developing a neural network retrieval, the SMAP study equally aims at investigating the potential of neural networks as a downscaling tool. Since they are able to model non-linear relationships, it is considered that a neural network-based downscaling algorithm could perform better than the linear SMAP downscaling techniques proposed so far.
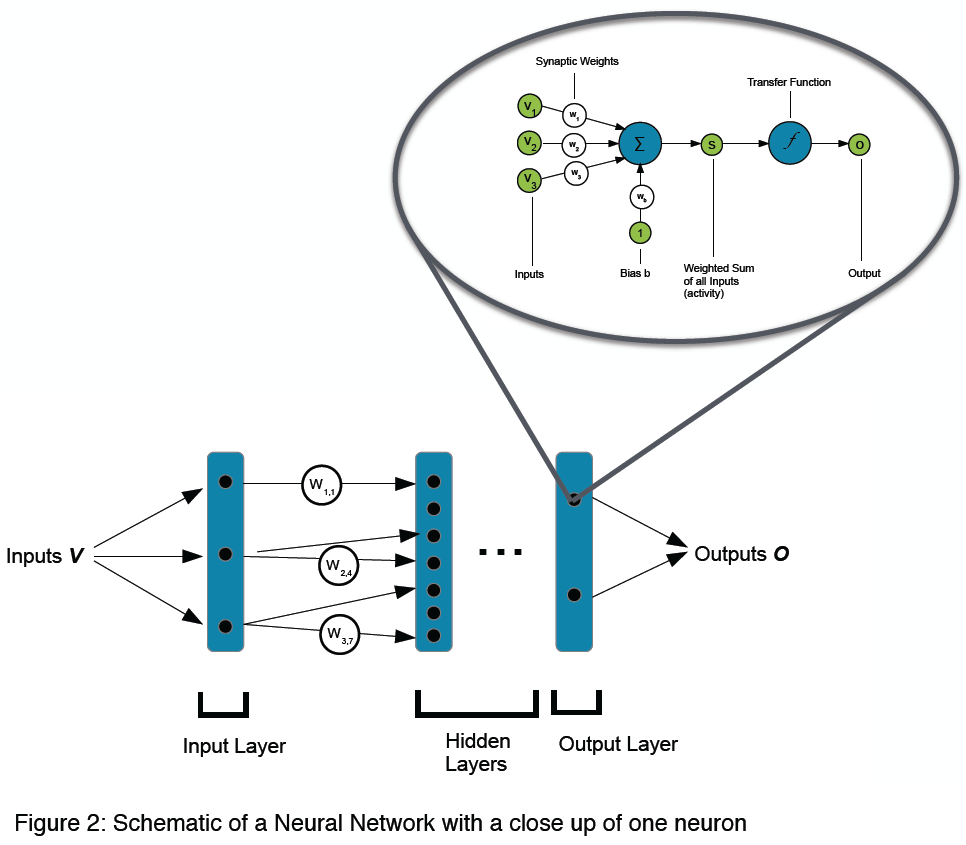
The neural network-based soil moisture retrieval is also greatly beneficial for the evaluation and improvement of both NWP as well as general land surface models. This is because soil moisture data retrieved using a neural network is compatible with the model used as part of the training process and hence, this data can be immediately assimilated into a model without any further calibration. Simplifying the data assimilation process facilitates the synergy of models and satellite data and helps improve the predictions made. In an alternative approach, the neural network based soil moisture dataset has also been used for a parameter-based evaluation of modeled soil moisture and has been found to be very useful in many areas. Finally, since neural networks model the relationship between different variables, they are useful tools to estimate the sensitivities between these variables. This means they can be used to compare the sensitivities found in models to those of observations and thus allow us to perform process-based model evaluations. This technique has been implemented for an analysis of the vegetation module of a land surface model, but is generally applicable to any modeled process.
*GCOS is a joint undertaking of the World Meteorological Organization (WMO), the Intergovernmental Oceanographic Commission (IOC) of the United Nations Educational Scientific and Cultural Organization (UNESCO), the United Nations Environment Programme (UNEP) and the International Council for Science (ICSU). Its goal is to provide comprehensive information on the total climate system, involving a multidisciplinary range of physical, chemical and biological properties, and atmospheric, oceanic, hydrological, cryospheric and terrestrial processes. It is built on the WMO Integrated Global Observing System (WIGOS), the IOC-WMO-UNEP-ICSU Global Ocean Observing System (GOOS), the UN Food and Agriculture Organization (FAO)-UNEP-UNESCO-ICSU Global Terrestrial Observing System (GTOS) and a number of other domain-based and cross-domain research and operational observing systems. It includes both in situ and remote sensing components, with its space based components coordinated by the Committee on Earth Observation Satellites (CEOS) and the Coordination Group for Meteorological Satellites (CGMS). GCOS is intended to meet the full range of national and international requirements for climate and climate-related observations. As a system of climate-relevant observing systems, it constitutes, in aggregate, the climate observing component of the Global Earth Observation System of Systems (GEOSS).
Project contact: Dr. Jana Kolassa